Aim and impact of BASE-II
Following the success of the BASE-I project, BASE-II will address identified challenges and requirements in developing AI for Science solutions at exascale. SciML bench, released as part of BASE-I, provided the scientific community with examples and templates for several challenging problems from different research areas. However successful, benchmarks alone cannot be the only solution for the AI for Science community’s challenges.
This work helped to formulate a core set of requirements gathered from the community, which we decided to concentrate on:
- Understanding the interactions between AI algorithms and systems across a range of scientific problems using benchmarks so that better AI software can be designed — with an emphasis on reproducibility (AI Benchmarking),
- Better HPC scalability using AI (AI/HPC Convergence)
- Cross-leveraging information about AI hardware and AI software for the betterment of the two through co-design principles (AI Hardware/Software Co-Design)
- Enabling scientists to understand better very large-scale, complex, and multi-modal datasets, with attention to incremental learning and generative modelling (Learning from Largescale datasets)
- Equipping the community with an ecosystem of tools, frameworks, and workflows to support the development of AI for Science applications for exascale (AI at Exascale Toolbox)
This project aims to develop a suite of exascale-ready software and relevant designs for addressing these highly prioritized requirements from the AI for Science community — Blueprinting AI for Science at Exascale.
We will ensure that our deliverables remain relevant to UKRI’s e-Infrastructures, and to the communities, through tight engagements with various ExCALIBUR-funded projects, industries, various user bases, academia, national laboratories, and international organisations. In addition, numerous knowledge exchange activities will underpin the maximum flow of information between relevant communities, leading to our success.
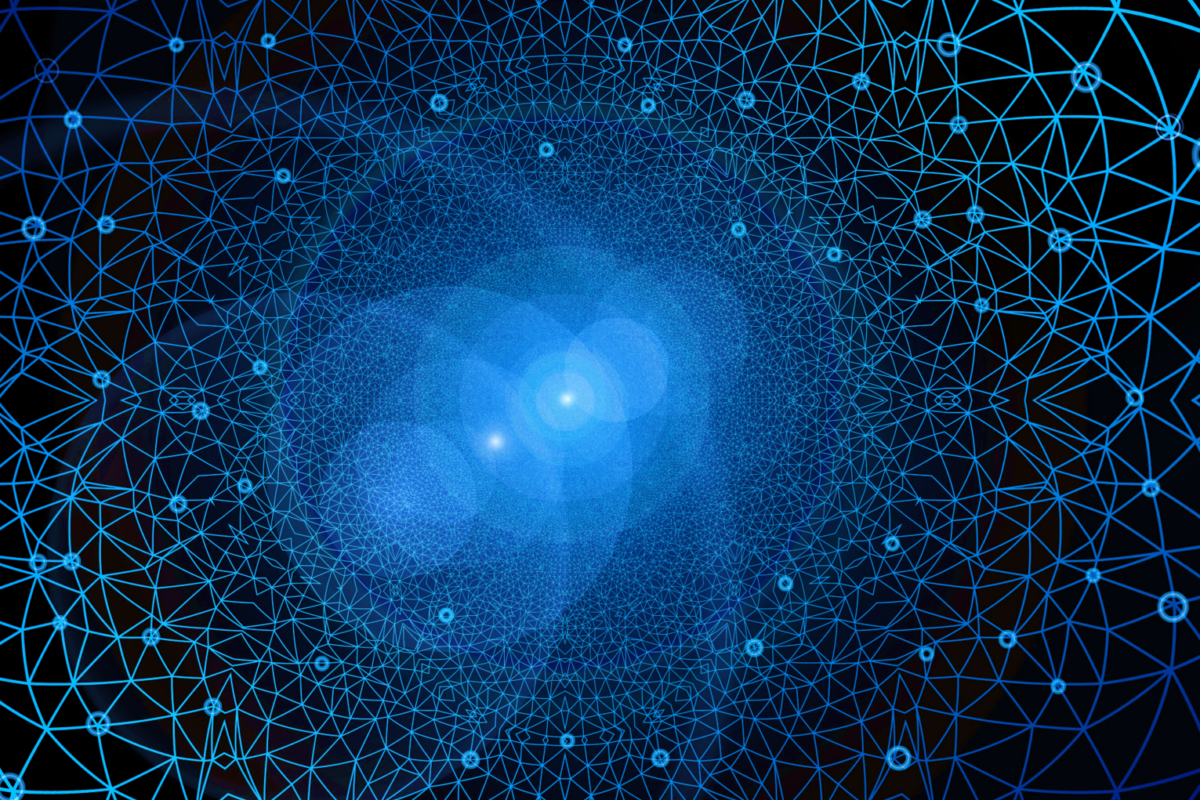